A Chinese research consortium has developed techniques to bring editing and compositing capabilities to one of the hottest image synthesis research sectors of the last year – Neural Radiance Fields (NeRF). The system is entitled ST-NeRF (Spatio-Temporal Coherent Neural Radiance Field).
What appears to be a physical camera pan in the image below is actually just a user ‘scrolling’ through viewpoints on video content that exists in a 4D space. The POV is not locked to the performance of the people depicted in the video, whose movements can be viewed from any part of a 180-degree radius.
Each facet within the video is a discretely captured element, composited together into a cohesive scene that can be dynamically explored.
The facets can be freely duplicated within the scene, or re-sized:
Additionally, the temporal behavior of each facet can be easily altered, slowed down, run backwards, or manipulated in any number of ways, opening the path to filter architectures and an extremely high level of interpretability.
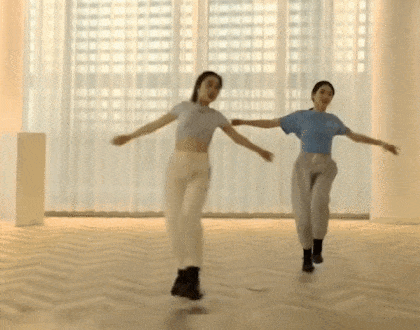
Two separate NeRF facets run at different speeds in the same scene. Source: https://www.youtube.com/watch?v=Wp4HfOwFGP4
There is no need to rotoscope performers or environments, or have performers execute their movements blindly and out of the context of the intended scene. Instead, footage is captured naturally via an array of 16 video cameras covering 180 degrees:

The three elements depicted above, the two people and the environment, are distinct, and outlined only for illustrative purposes. Each can be swapped out, and each can be inserted into the scene at an earlier or later point in their individual capture timeline.
ST-NeRF is an innovation on research in Neural Radiance Fields (NeRF), a machine learning framework whereby multiple viewpoint captures are synthesized into a navigable virtual space by extensive training (though single viewpoint capture is also a sub-sector of NeRF research).

Neural Radiance Fields work by collating multiple capture viewpoints into a single coherent and navigable 3D space, with the gaps between coverage estimated and rendered by a neural network. Where video (rather than still images) is used, the rendering resources needed are often considerable. Source: https://ift.tt/3gGztDX
Interest in NeRF has become intense in the last nine months, and a Reddit-maintained list of derivative or exploratory NeRF papers currently lists sixty projects.

Just a few of the many off-shoots of the original NeRF paper. Source: https://ift.tt/3exNjKj
Affordable Training
The paper is a collaboration between researchers at Shanghai Tech University and DGene Digital Technology, and has been accepted with some enthusiasm at Open Review.
ST-NeRF offers a number of innovations over previous initiatives in ML-derived navigable video spaces. Not least, it achieves a high level of realism with only 16 cameras. Though Facebook’s DyNeRF uses only two cameras more than this, it offers a far more restricted navigable arc.
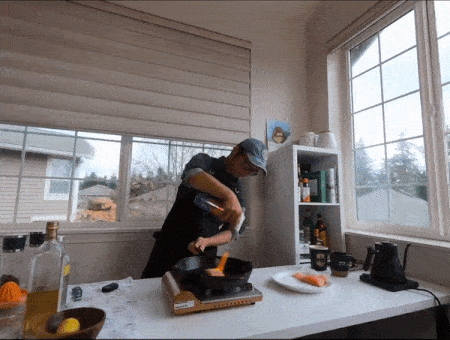
An example of Facebook’s DyNeRF environment, with a more limited field of movement, and more cameras per square foot needed to reconstruct the scene. Source: https://ift.tt/2OgzvJw
Besides lacking the ability to edit and composite individual facets, DyNeRF is particularly expensive in terms of computational resources. By contrast, the Chinese researchers state that the training cost for their data comes out somewhere between $900-$3,000, compared to the $30,000 for the state-of-the-art video generation model DVDGAN, and intensive systems such as DyNeRF.
Reviewers have also noted that ST-NeRF makes a major innovation in decoupling the process of learning motion from the process of image synthesis. This separation is what enables editing and compositing, with previous approaches restrictive and linear by comparison.
Though 16 cameras is a very limited array for such a full half-circle of view, the researchers hope to cut this number down further in later work through the use of proxy pre-scanned static backgrounds, and more data-driven scene modeling approaches. They also hope to incorporate re-lighting capabilities, a recent innovation in NeRF research.
Addressing Limitations of ST-NeRF
In the context of academic CS papers that tend to trash the actual usability of a new system in a throw-away end paragraph, even the limitations that the researchers acknowledge for ST-NeRF are unusual.
They observe that the system cannot currently individuate and separately render out particular objects in a scene, because the people in the footage are segmented into individual entities via a system designed to recognize humans and not objects – a problem that seems easily solved with YOLO and similar frameworks, with the harder work of extracting human video already accomplished.
Though the researchers note that it is currently not possible to generate slow-motion, there seems little to prevent the implementation of this using existing innovations in frame interpolation such as DAIN and RIFE.
As with all NeRF implementations, and in many other sectors of computer vision research, ST-NeRF can fail in instances of severe occlusion, where the subject is temporarily obscured by another person or an object, and may be difficult to continuously track or to accurately re-acquire afterwards. As elsewhere, this difficulty may have to await upstream solutions. In the meantime, the researchers concede that manual intervention is necessary in these occluded frames.
Finally, the researchers observe that the human segmentation procedures currently rely on color differences, which could lead to unintentional collation of two people into one segmentation block – a stumbling block not limited to ST-NeRF, but intrinsic to the library being used, and which could perhaps be solved by optical flow analysis and other emerging techniques.
from Hacker News https://ift.tt/3xRbrz7
No comments:
Post a Comment
Note: Only a member of this blog may post a comment.